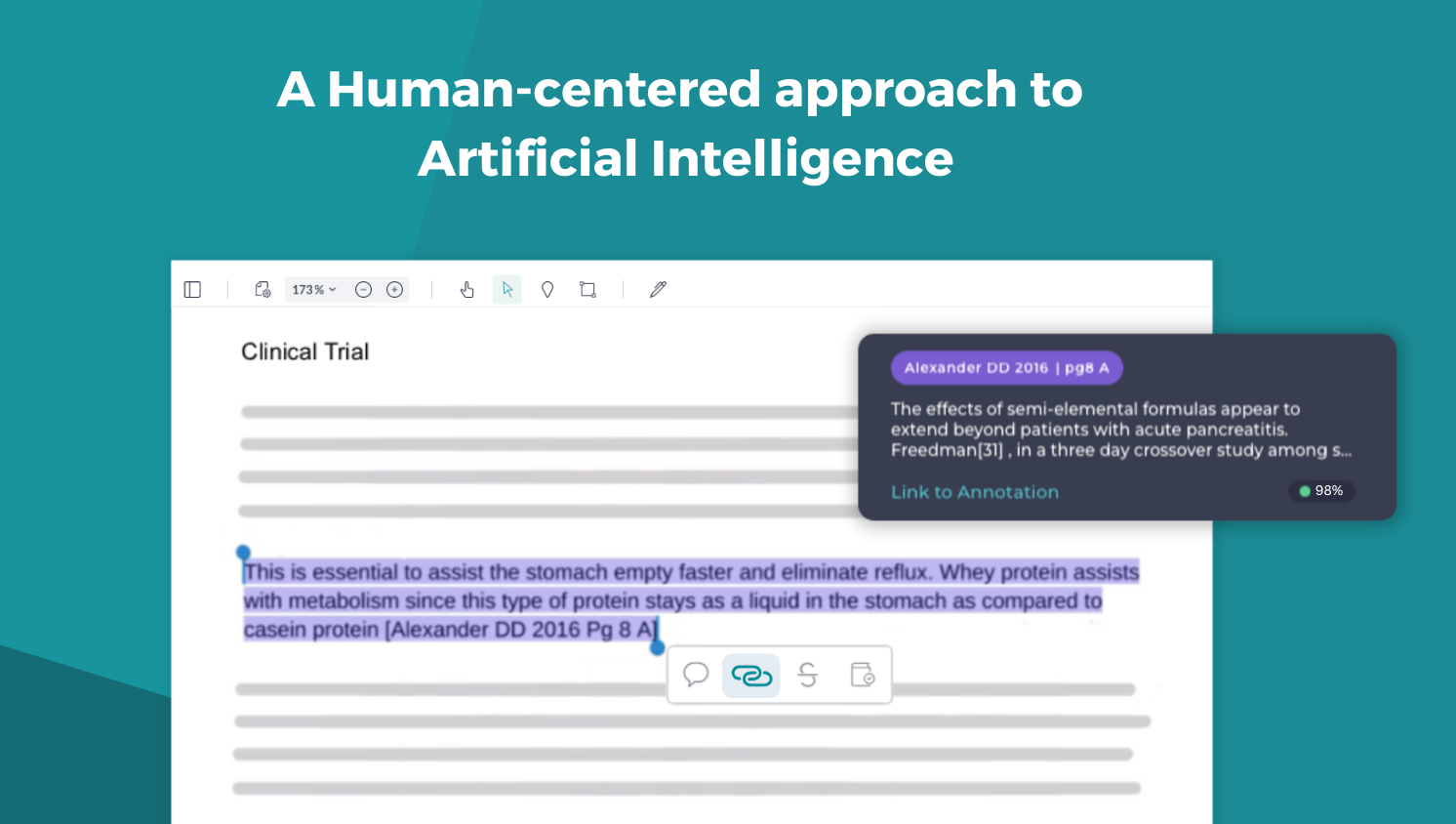
A Human-centered approach to Artificial Intelligence
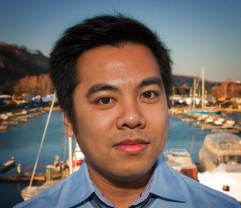
Overview
Papercurve’s platform began as a modern, user friendly document management system tailored for the life science and pharmaceutical industry.
Our platform allowed teams from life science companies to collaborate on promotional material, speed up regulatory review, and manage contracts with healthcare professionals.
We were starting to accumulate a lot of content from our users. From drug detail aids, scientific platforms, product monographs, we had an opportunity to begin developing Papercurve’s AI strategy but our approach to AI needed to be different.
The problem with AI
It seems that AI is everywhere. Everyone talks about how it’s the next revolution in our technological advancement and for the most part AI has been and will be providing new efficiencies and productivity we have not seen before.
However, there is a big problem when navigating the AI landscape trying to find the right solution for you, your team, and your unique use case.
That is most AI strategies rely on three key pillars:
1. Big Data
2. Automation
3. Generative AI.
Big Data
The race to have the biggest data models are coming to an eventual end. Technologies have reached a point where they’ve consumed a large entirety of the internet and are able to help you generate marketing copy or have human-like conversations with a chatbot.
The problem here is that the big data models are trained on generally available content. With our clients being in the life sciences and pharmaceutical industries, the data that is relevant is private, proprietary, and unique to the company and their teams.
Big data models are filled with irrelevant information for your needs.
Automation
A lot of technology providers will promise the world in fully automating your promotional content creation. This is especially difficult with content pertaining to life sciences and pharmaceuticals.
The words within the content are dense with scientific terms, specific situations, and many edge cases where a human is still the best decision maker.
The promise of difficult content decisions being made by technology is very appealing, but we are not there yet.
Generative AI
Generative AI has a truth and factuality problem, but it has great confidence in its inaccuracy.
Since it requires big data sets to be able to generate content, it often cannot tell what the real truth is, and instead provides information that it believes is true, but may not actually be the case.
This is highly problematic in life sciences and pharmaceuticals.
If generative AI is to work, it will have to be trained from your data and your team’s data alone.
Understanding the AI landscape is key to develop a useful AI strategy.
At Papercurve, we understand that your problems are unique, your teams are unique and your data is unique.
With this in mind, our teams knew that our solutions would have to be just as unique, - and we started from scratch.
The Beginnings of a Strategy
The development team at Papercurve came together to identify a few rules.
1. First, since our client's content is private and unique to their own use case and team, the AI strategy would always need to start from scratch. This means that the first rule is that no data will be shared between clients and the solution would grow organically from nothing as more users interact with their team’s documents on their own customized Papercurve platform.
2. Second, since the teams dealing with content are typically small, the amount of data we needed to work off of is not in the realm of what normal machine learning was used to dealing with. So whatever solution we came up with, we had to work within the limitations of small data since big data was not a possibility with small teams.
3. Third, knowing the industry and the fixation with AI solutions, we knew there were a lot of software vendors not providing the real value and utilizing their AI strategy more as a marketing gimmick than an actual value-added solution. So, the Papercurve engineering team decided to have a focus on one key metric to make sure we were on the right path to providing value with our AI solution.
The key metric was review time.
Since a lot of the documents on our platform were being processed through promotional and regulatory review, this was one of the metrics that encompasses a goal that will benefit our users.
Papercurve allows collaboration with your teams easily and intuitively.
We wanted to leverage the way users were interacting with their documents, so we designed features with our AI strategy in mind.
We provide tools to comment, create references, and to classify textual content within documents.
When users take action on content within documents, we record dozens of data points in the interaction to help our training.
Identifying text through reference classification, product claims, marking up removals, identifying modifications, we were well on our way to start training and testing results.
In order to ensure we are going on the right path; the Product team would interview and analyze the data from key partners that have agreed to be beta testers of our new path to AI.
As part of our DNA, our core value is that Software Disappears.
We often describe this to our clients because it is a vital part of how we create software and solutions at Papercurve.
Software disappearing means the user is not burdened by hours of training to learn how to use it, the user interface and experience is intuitive enough to allow anyone to understand it.
Most importantly, software disappearing means you are not being blocked by platform and are focused on what your task at hand is.
By having rigid core values and listening to our customers closely, we were able to design an AI strategy that actually delivered value.
And with that our team developed:
Papercurve’s Paige AI - Our Human-centered approach to AI
How it works
With our strategy now in place, the stage was set. However, we’d have to solve some technical barriers.
Even though we were gathering all this data, AI needs a lot of data to have some certainty or a decent level of confidence.
Our engineers spent about 6 months developing an in-house machine learning strategy that would be able to predict the content with a high level of accuracy and confidence.
We knew that our limitation of not having big data would be a difficult technical challenge, but the team was able to develop through iterative experimentation and testing, a way to leverage small data to deliver big results and high confidence scores.
Since we had a strong machine learning strategy powered by months of developing the right tooling, we began identifying some key classifications and interactions on the platform. We analyzed 12,000 collaborative comments from our platform and found out what most comments required. The majority of comments stemmed from specific modifications, the second highest number of comments referred to questions and clarifications.
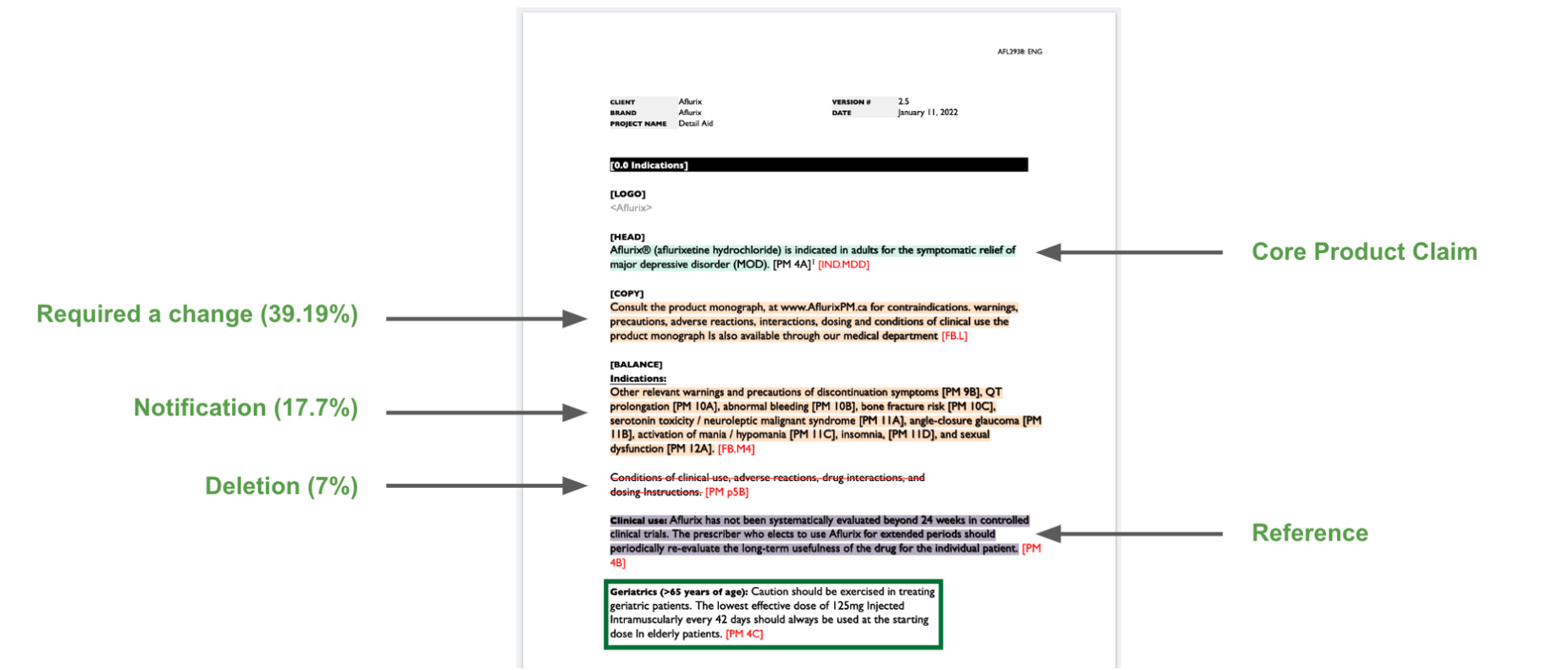
One of the most utilized annotation tools is with regards to making references. When a medical claim is made in a piece of content, evidence to back up that claim is linked to external reference documents.
This allows reviewers to look at the evidence and make a judgement to approve the content based on the reference. Giving reviewers context is critical to a speedy review and approval.
We now had a strategy, customized machine learning tools to execute training our AI, and most importantly enough data to start executing.
We shortly released References powered by Paige AI. By specifically designing features on our platform with our AI strategy in mind, teams that use our platform were already classifying content and helping gather data for Paige to train on.
The Results
After the first release of References powered by Paige AI, we wanted to ensure we created a solution that provided real value to our users. From a very standard usage of a small team, Papercurve’s Paige AI was able to make recommendations with high confidence scores with limited data sets.

We analyzed Referencing on the Papercurve platform with and without our Paige AI solution and found that it was able to speed up reference creation by 10x. This increased the time to approval on promotional review content allowing our users get to regulatory readiness quicker and with less errors.
The Next Generation
With the success of references, we wanted to iterate on the solution more. Since Product Claims were also a way to classify content, we implemented a similar solution as what we did for references.
The product development team wanted to go a step further.
Now that we were able to find and identify content and properly classify them accurately, we tested different ways to surface these recommendations to the user without it being intrusive.
We tested dozens of user flows to be able to find the right experience. Recommendations from AI have to be trained with the assistance of the user’s inputs and the findings from that have to be handed to the user the moment they need it.
Papercurve’s design team tries to create magic moments in order to improve the experience of our users.
For product claims, we not only provide features to surface pre-approved claims when trying to stay on message with your brand and avoid regulatory burdens for re-approving old content, we surface these recommendations when you most likely need them.
When commenting on textual content that you know is not the correct wording, Product Claims powered by Paige AI surfaces any problems it sees with the content based on previously approved claims.
This allows users to stay on message, avoid regulatory approval burdens on already used previously approved content and speeds up the review time by not having to manage a claims book via spreadsheet doing manual lookups.
Since our technology is not based on exact text matching but actual natural language processing, we are able to be more flexible with identifying errors with similarly worded phrasing.
Going Forward
We have only begun our AI rollout, our product and engineering teams are hard at work on our biggest releases coming up.
We will be introducing Papercurve Office.
Fully capable cloud editing on the Papercurve platform compatible with Microsoft Office formats.
This has been an upgrade we’ve been planning on for over a year and it allows us to unlock Paige AI’s capabilities within a document editor.
With Papercurve Office, we will be able to analyze and identify references, product claims, and even errors as our users build content on the platform.
All ensuring that we help increase value to our customers by unlocking efficiencies in the promotional content lifecycle.
Our Purpose Drives Everything
After the first few years of Papercurve, we’ve realized that our purpose:
To accelerate the connection between the patient and life enhancing treatments.
It is what drives our decisions at Papercurve and how we stay on the right path when developing software and AI solutions. Because of this purpose and our approach to creating software, we found that time to “Everyone Approved” improved by an average of 36% after the first quarter of utilizing Papercurve’s platform.
With the creation of our human-centered approach to AI philosophy, we can provide much needed value in such an important space.
📅 Come see our CTO speak more about this topic at Collision in Toronto on June 28th!
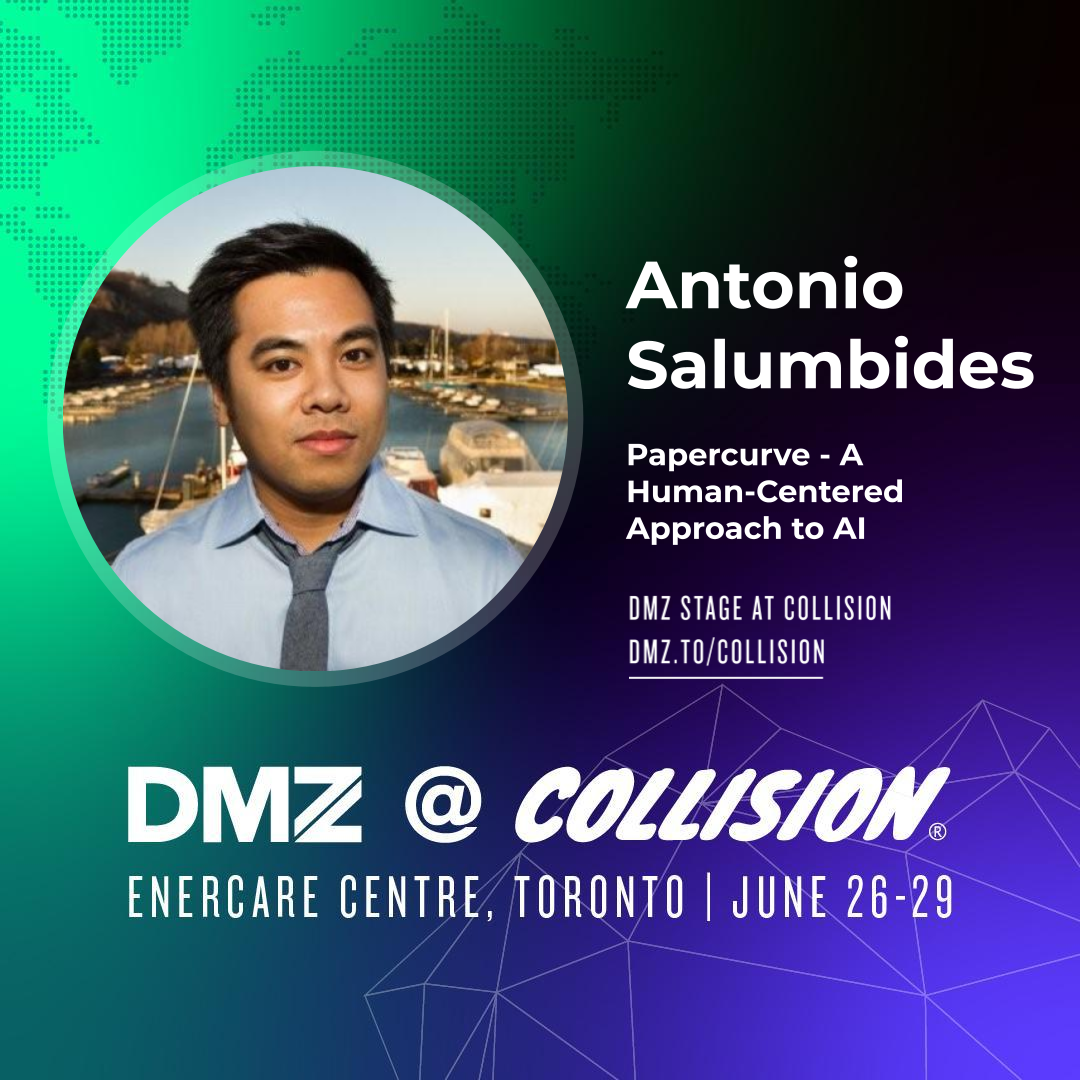