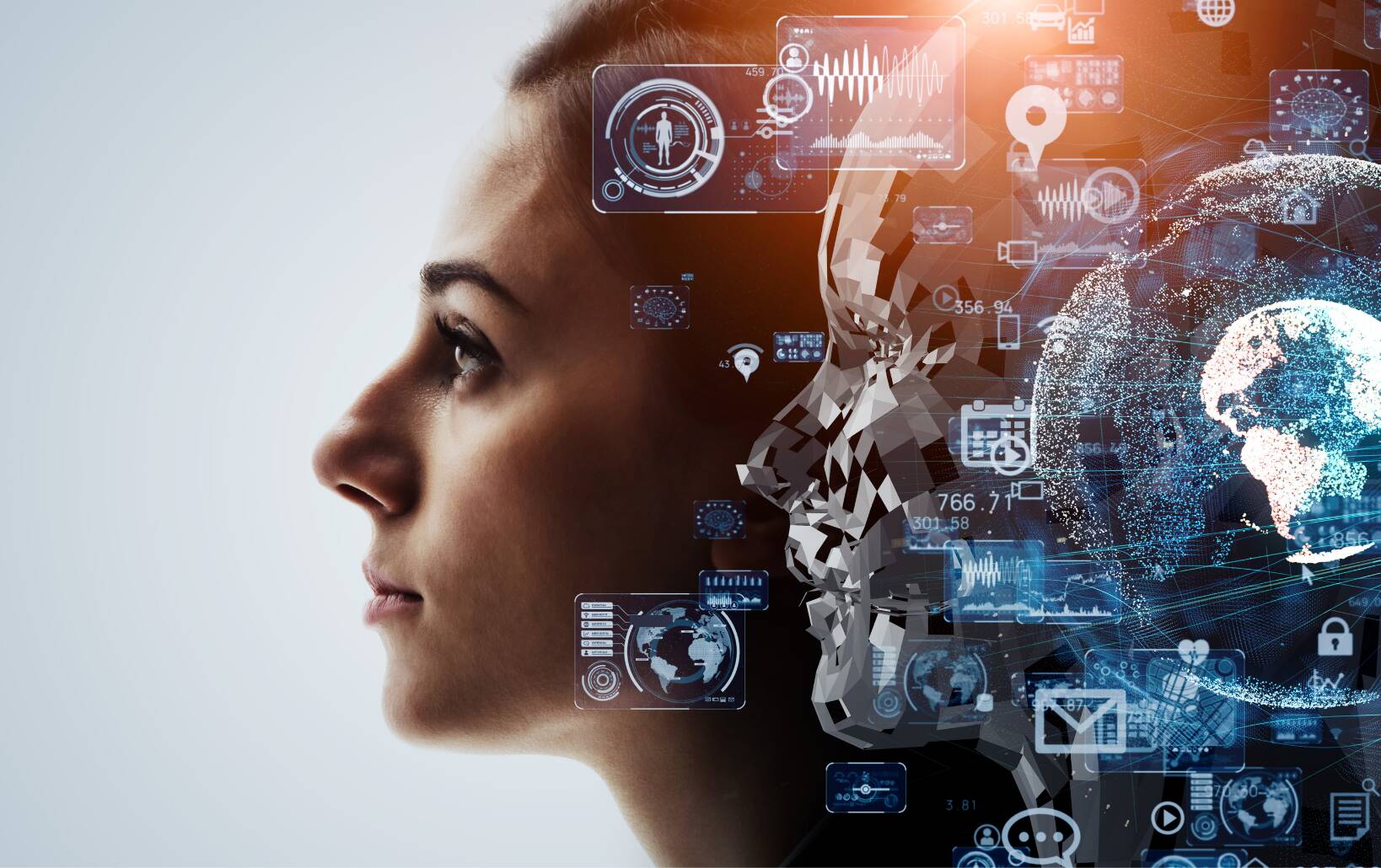
How our AI Recommendations Improve over time with Training
We've all heard that "practice makes perfect."
There is no such thing as a born expert. Instead, the more we practice, the better we become. It is how we learn and progress: through training our brains. Just like humans need training to develop their skills, machine learning works in the same way. It acquires knowledge through training. Machine learning can be taught and excel at tasks that can be repetitive and time-consuming for humans. AI is transforming medical affairs and commercial teams.
Let's take Paige AI: Assisted References as an example.
Paige AI: Assisted References, Papercurve’s AI assistant, and other machine learning applications operate like a human brain that learns and becomes smarter over time. Paige AI links text in a document with a reference previously created, making the referencing process 10x quicker. Linking a reference every time is slow and difficult to grasp. Paige AI solves these challenges by making referencing faster, easier, and more accurate. The more references that are annotated, the more accurate the recommendation will be.
How accurate are recommendations after linking a reference one time?
The first step to training Paige is to create a reference manually. Then, Paige uses this information to recommend the reference in the future accurately.
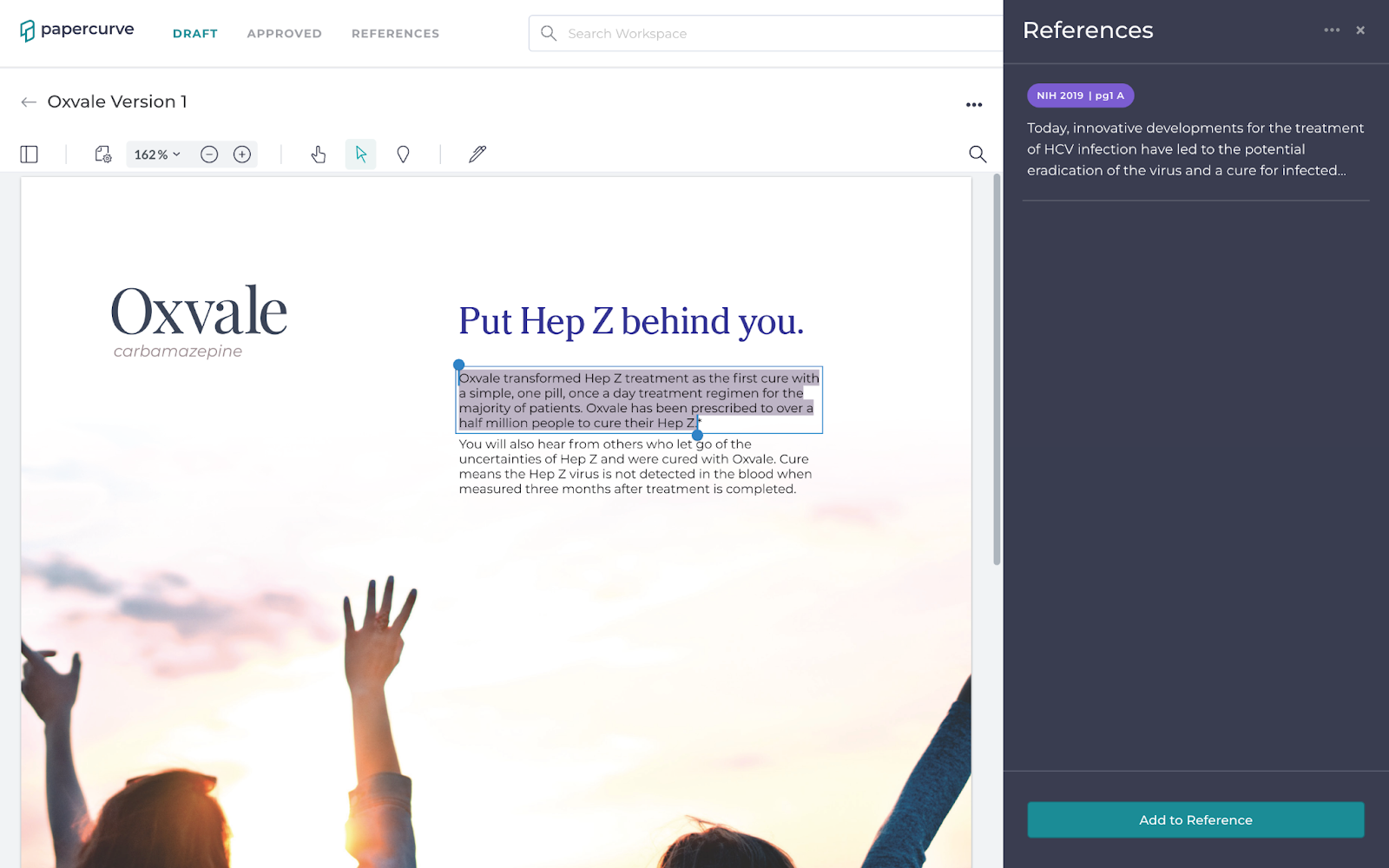
Paige is being trained to associate this paragraph “Oxvale transformed Hep Z…” with a reference that has the label “NIH 2019 Pg 1 A”.
If this paragraph is used in the future, Paige will suggest the correct reference, which can be linked with a click. Paige correctly suggested the “NIH 2019 Pg1 A” reference with a confidence score of 84%. The more Paige AI is trained, the more accurate the recommendations. Paige will continue to improve its ability to recognize patterns and interpret references.
In the example above, Paige’s capabilities are based on a previously linked reference, and the confidence score was high because the text was identical. It illustrates the power of machine learning even with limited data.
If the text were reworded, what would Paige's reaction be?
If the text above is changed, Paige would recognize the intent and suggest the correct reference. In this example, Paige recommended the proper reference with an 80% confidence score even though the text was partially different.

Paraphrasing the same text and using it in a different context is common. Paige will recognize the message and provide the appropriate reference if the message is the same. As Paige gains more experience, it will recognize these claims. Here’s where you can see more examples of How Natural Language Processing improves Reference Creation.
Discover how Paige AI can help accelerate referencing and reduce repetitive, time-consuming review tasks.